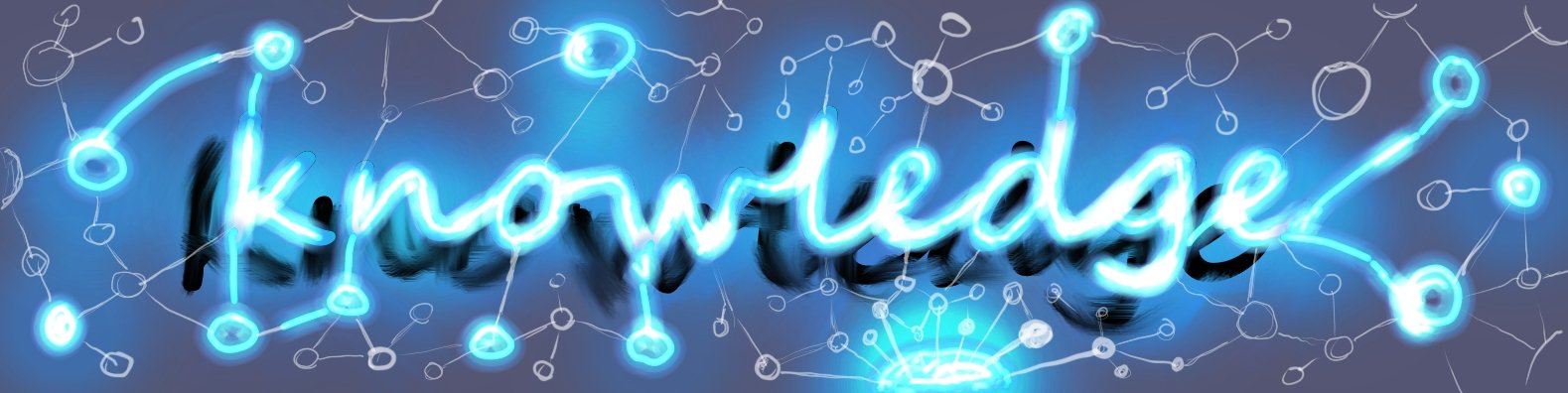
Vectors & Graphs
Ontologies and Knowledge Graphs offer a way to connect embedding vectors to structured knowledge, enhancing their meaning and explainability
The Great Compression
We are witnessing an era of information compression, spearheaded by large language models (LLMs) that proficiently process web text. These LLMs handle an inconceivably vast array of word combinations, reducing them to a mere trillion parameters. Embedding models, like text-embedding-ada-002, further condense this into 1536 dimensions. Reflect on this when using Retrieval-Augmented Generation (RAG): the essence of the web's information, distilled into 1536 coordinates.
Data Connectivity and the Free Energy Principle
Think of your organisation as a living entity—its survival depends on a well-defined information boundary, which functions like a semi-permeable membrane. Karl Friston's Free Energy Principle (FEP) models this boundary as a Markov Blanket and says that to sustain itself, a system must minimise its free energy.
Continuous and Discrete
We can think of information existing in a continuous stream or in discrete chunks. Large Language Models (LLMs) fall under the category of continuous knowledge representation, while Knowledge Graphs belong to the discrete realm. Each approach has its merits, and understanding the implications of their differences is essential.
How Ontologies Can Unlock LLM for Business
This post delves into the transformative capabilities of Large Language Models, such as GPT-4, and examines the crucial role that the structured intelligence of ontologies can play in deploying LLMs in production environments.
Graph of Thought
Imagine the next phase: a ‘Graph of Thought’ where thoughts are modelled as nodes connected by edges. Directed Acyclic Graphs (DAGs) have revolutionised data pipeline orchestration tools by modelling the flow of dependencies in a graph without circular loops. Unlike trees, DAGs can model paths that fork and then converge back together! This is a game-changer, and surely it's only a matter of time before LLM thought prompting embraces this powerful ability too.
LLMs + Ontologies
This collaborative partnership between LLMs and ontologies establishes a reinforcing feedback loop of continuous improvement. As LLMs help generate better ontologies faster and more dynamically, the ontologies, in turn, elevate the performance of LLMs by offering a more comprehensive context of the data and text they analyse
How do you make your data more intelligent?
Concentrate on the fundamentals first. Don’t get distracted by side projects. AI needs data! You can now buy general intelligence, but only you can provide your private data. However, every organisation's data is currently disconnected and poorly organised. If you want to use all this intelligence in a way that is meaningful to your organisation, then you must first get your data into a shape that is ready for use with AI.
Your Ontological Core
There are pragmatic techniques organisations can use here: they can connect their data by giving each data point a URL and structure their data by linking these URLs to concepts in shared ontologies. Linked Data compresses very well, and you can use it to finetune a private model that reflects your organisation's identity. This is your solid core. Your solid core projects out holographically onto the surface boundary of your organisation, and in an AI-driven world, it will increasingly come to define you.
Data, Graphs and AI
In an enterprise context, AI models unlock their true potential not merely through the vast quantities of information they process, but when that information is relevant, connected, cleaned, structured, and enriched with semantics—all tasks that lie at the heart of data management expertise. Yet, the reverse is equally true: our data strategies gain direction and sophistication when guided by the insights and capabilities AI brings to the table. Moreover, AI can help you connect, clean, structure, and enrich your data with semantic metadata.
Network of Networks,
Taking control of how you prepare your data gives you agency. The key to preparing your data for AI is enhancing its compressibility. This involves focusing on the relationships between data items, not just the items themselves, because better connectivity equals better compressibility.
You can add meaningful connections to your data in two main ways:
Data Products + Ontologies
Many organisations are reorganising their data around data products, and the most advanced are connecting their data with knowledge graphs for use with Gen AI. These efforts should not be separate endeavours; the real value lies in bridging them.
This is where DPROD comes in. It is a freely available semantic ontology that defines data products, serving as both a specification and a first small step in creating a Distributed Knowledge Graph.
LLMS Love Graphs
As we move into the next phase of AI adoption, where organisations must feed their own data into large language models to realise their benefits, it's crucial to model the relationships explicitly. Large language models thrive on these relationships; that's where the power lies.
The Semantic Layer
By employing these open technologies and standards, any organisation can construct a Shared Semantic Layer, which gives a uniform and consistent understanding of data. Most importantly, this makes technologists consciously think about designing data products in a way that directly delivers business value in business terms
The Semantic Router
Here's the idea: the router initially maps the question to relevant classes within the organisation's upper ontology—a structured representation of key concepts that your business is focused on. Utilising these classes, the router then retrieves the corresponding 'Semantic Data Product' from the organisation's Semantic Layer
Human In The Loop
Knowledge Graphs and Ontologies provide the sophisticated tools required to articulate our complex desires and ethical frameworks. They offer a robust mechanism for anchoring humanity at the critical juncture where fluid information crystallizes into discrete units. By weaving thoughtful and considered human insight into this AI feedback loop, we're not just making incremental improvements; we're laying the architectural groundwork for a more enlightened form of artificial intelligence.
The Working Memory Graph
To build a WMG, the LLM processes a question and returns a graph of nodes using URLs as identifiers, these URLs link to ground truths stored in the organisation's Knowledge Graph. The WMG can also incorporate nodes representing conceptual understanding, establishing connections between the LLM's numerical vectors and the KG's ontological classes.
Reinventing The Wheel
So let's get Data Mesh and Data Contracts right, by building them upon the solid foundations provided by Knowledge Graph technology. Let’s reinvent the wheel in the right way, by founding it upon a proven technology that honours interconnectivity.
Seeing The Big Picture
Some of us are talking about Data Meshes, while others are talking about Semantic Layers and yet another group is talking about Enterprise Search etc. I can’t help wondering if we are all just talking about different aspects of the same thing. When each aspect is connected they combine to form one thing: a Knowledge Graph.
Transformers and GNNs
Transformers analyse sentences by assigning importance to each word in relation to others, helping them predict or generate the next words in a sentence. This 'attention mechanism' evaluates pairwise interactions between all tokens in a sequence, and these interactions can be seen as edges in a complete graph. Thus, Transformers can be thought of as graph-based models where tokens represent nodes and attention weights represent edges
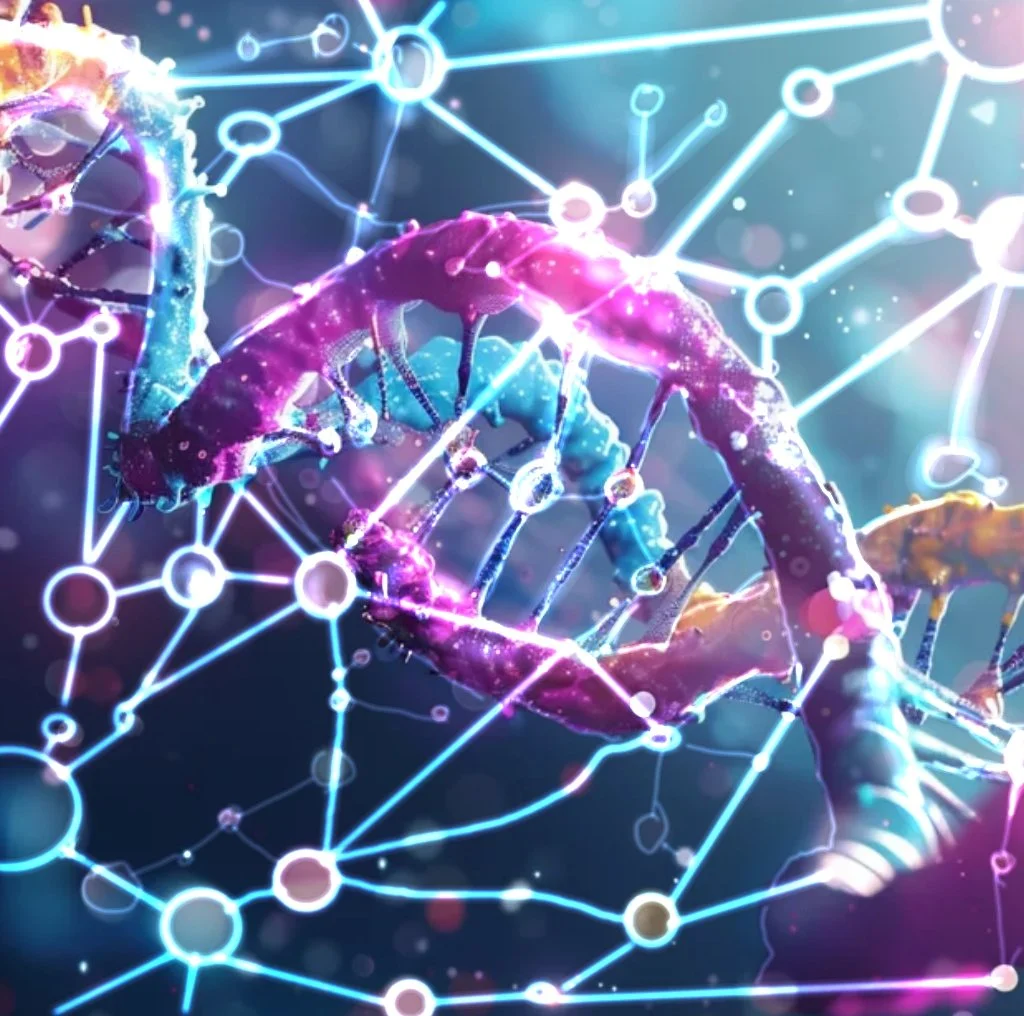